Flex StaffingPlus
A seamless, integrated solution that creates a better experience
for Flex customers and their crews.
Start your free trial today!
YOUR
SUCCESS
IS OUR

Cloud Based Inventory Management Solutions
Why Flex?
Because "good enough" wasn't.
Our cloud-based inventory management and rental software solution is built upon our decades of experiences in the live event industry. We are the first cloud-based rental software created from the ground up for the event industry. We knew our industry couldn’t do its best work without the best platform. Hacking together the bits and pieces of other platforms not only didn’t sit right, but also it didn’t work. So we created Flex. For you. Our success is your success.Your Success is Our Flex.
See What Our Customers Say
Customer Spotlight: Legal Media Inc (LMI)
Meet Legal Media When we ask you to imagine a live event, we’re guessing that most of you easily conjure up images of concerts, sporting events, awards shows, and corporate banquets. But, did any of you think about a courtroom? We’re guessing no. But, when you stop and think about it, what’s more “live event”…
Read MoreCustomer Spotlight: Nighthawk Video
Meet Nighthawk Video Nighthawk Video is born from innovation. Its’ DNA can be traced to the early 1980s when Herbie Herbert, the manager of Journey, using video screens & cameras changed the Rock & Roll concert experience forever. Prior to Herbie & Journey pioneering live video, concerts were purely an audio, stage set and lighting…
Read MoreGo BTS and See FlexCon ‘23 Being Built in Flex!
In our OPAV Spotlight we promised a behind-the-scenes look at the making of FlexCon ’23. OPAV’s Tony Brinkman gives us all a quick Flex Master Class as he runs through the basics of setting up our event. Ready to see what Flex Rental Solutions can do for your business? Learn More About Flex Rental Solutions It’s easy…
Read MoreCustomer Spotlight: OPAV
Meet OPAV As the technical director for Orlando-based OPAV, a live event production company, with specialized experience in nationwide end-to-end production, Ian Paul is a damn nice guy. We were fortunate to spend a few minutes with him the day after he celebrated his ten-year anniversary with the fifteen-year old company. A Flex customer since…
Read MoreCustomer Spotlight: Pynx Productions
Meet Pynx Productions Not gonna lie. This might have been one of our favorite spotlight interviews to date. Not only because Mike D’Eri, owner and CEO of Canada-based production company, Pynx Productions, had amazing things to say about Flex. And not only because Mike tried one of our competitors and the onboarding alone was such…
Read MoreCustomer Spotlight: Quest Audio Visual
Meet Quest Audio Visual As we spoke with Ryan Peddigrew, director of business development for Toronto-based Quest Audio Visual, there were a few key figures that popped up: – 10-year Flex customer – 1,000,000 + scans – 10K+ events – 15+countries Still, there was one number that really stood out: 1. As in…
Read MoreCustomer Spotlight: Hardin-Simmons University
Meet Hardin – Simmons University The old saying goes, “Everything’s bigger in Texas.” After speaking with Zachary McNair, on Hardin-Simmon University’s Media Services Team, we’re inclined to agree. Only instead of steaks or maybe landmass, what’s bigger about HSU is its heart If you haven’t heard of Hardin-Simmons University, it is described as a “Baptist…
Read MoreCustomer Spotlight: EPiQVision
We’re not exactly sure how else to start this piece about Toronto-based A/V production house, EpiQVision, except to say that President/Owner, Jordan Benson, is a genuinely good dude. We’re proud that all of our customers are good people (they are, after all, in the business of creating memorable experiences that make our lives better), but…
Read MoreCustomer Spotlight: Firehouse Productions
“From the beginning it has been all about the best. It wasn’t about anything more. It wasn’t about anything less.” That’s how Brian Olson, founder of New York-based Firehouse Productions, set the tone for the company. It’s also how Mark Dittmar, Vice President of Sales, set the stage for our conversation. As Flex was founded…
Read MoreCustomer Spotlight: Show & Design Group
The Show & Design Group success story begins where many begin – in a spare bedroom with a few bits of this, a little of that, not much money, and the nagging feeling that something bigger and better was waiting to be built. That was then (2015). And as principal owner, Lew Aronoff recently shared…
Read More
Why use Flex's Cloud Based Inventory Management? Because we scale as you scale
With powerful features like inventory scanning, location tracking, availability viewing, labor scheduling, and quoting and invoicing, Flex Rental Solutions was custom designed to support the unique needs and challenges of live event, media production, and AV rental businesses and more.
Regardless of your industry, business model, or the unique angle your organization approaches problem solving from – we've seen it before. And if we haven't? Bring it on. Got something harder? Try us

Let's do it.
Reach out to us now, and let us know what you're looking to achieve.
Benefits to Our Cloud Based Rental Software
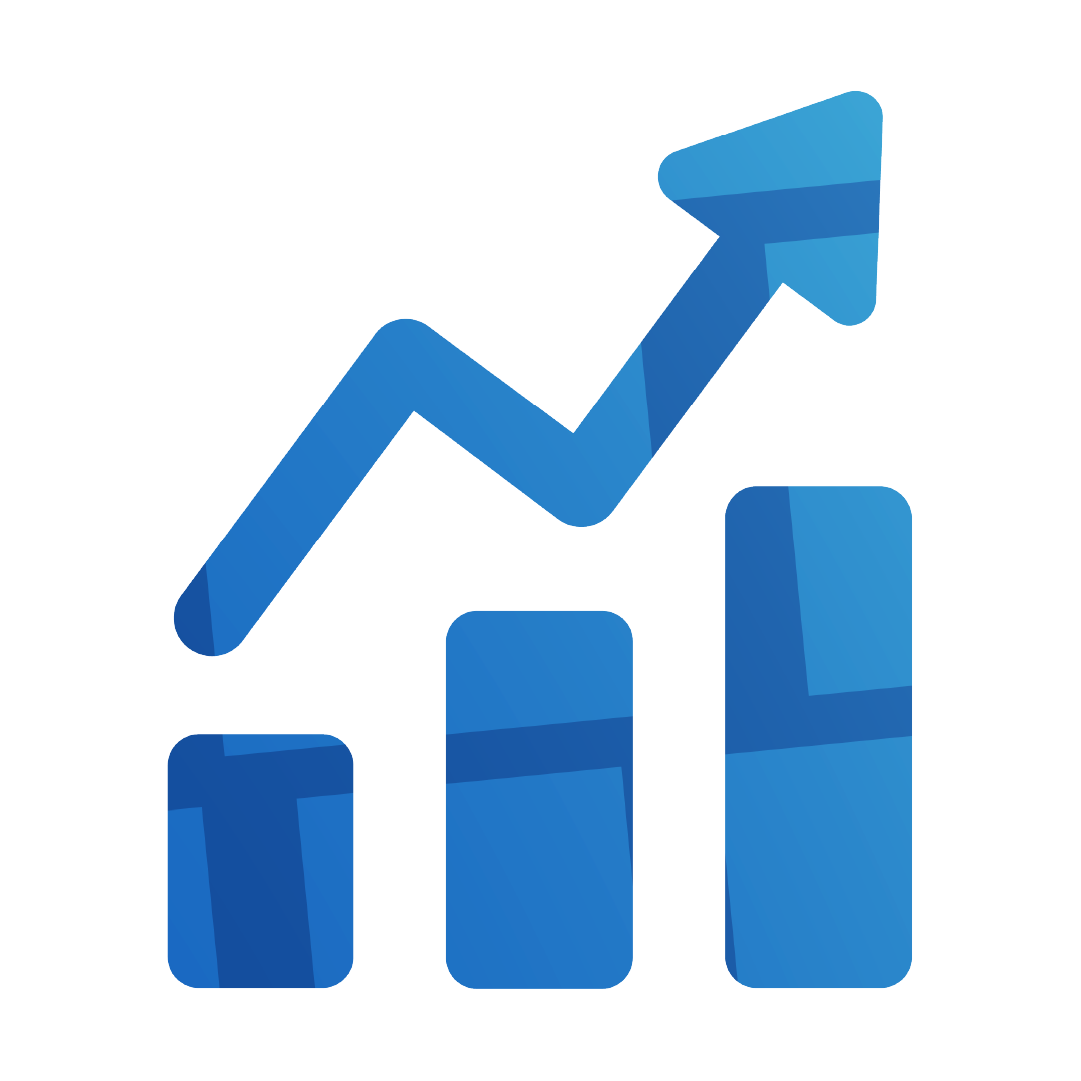
Increase ROI
Flex will have an immediate impact on your bottom line. Better asset management creates more revenue opportunities. You’ll produce better estimates. Accounts payable will be more efficient. Your assets will work harder for you. And that’s just for starters
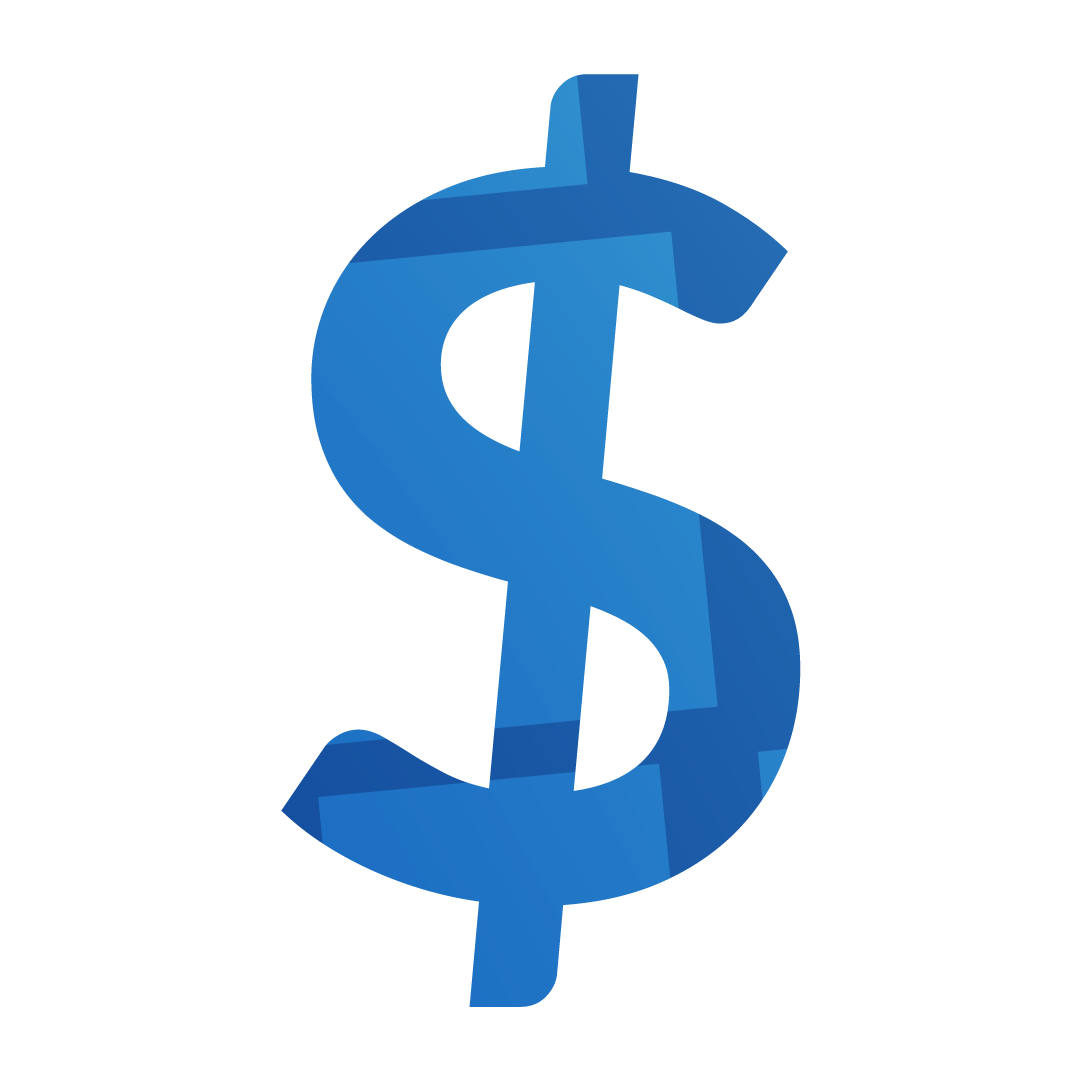
Reduce Cost
Cutting down on loss reduces costs. Faster training reduces costs. Retention reduces costs. Identifying and removing redundancies reduces costs. Flex helps reduce costs across your organization.
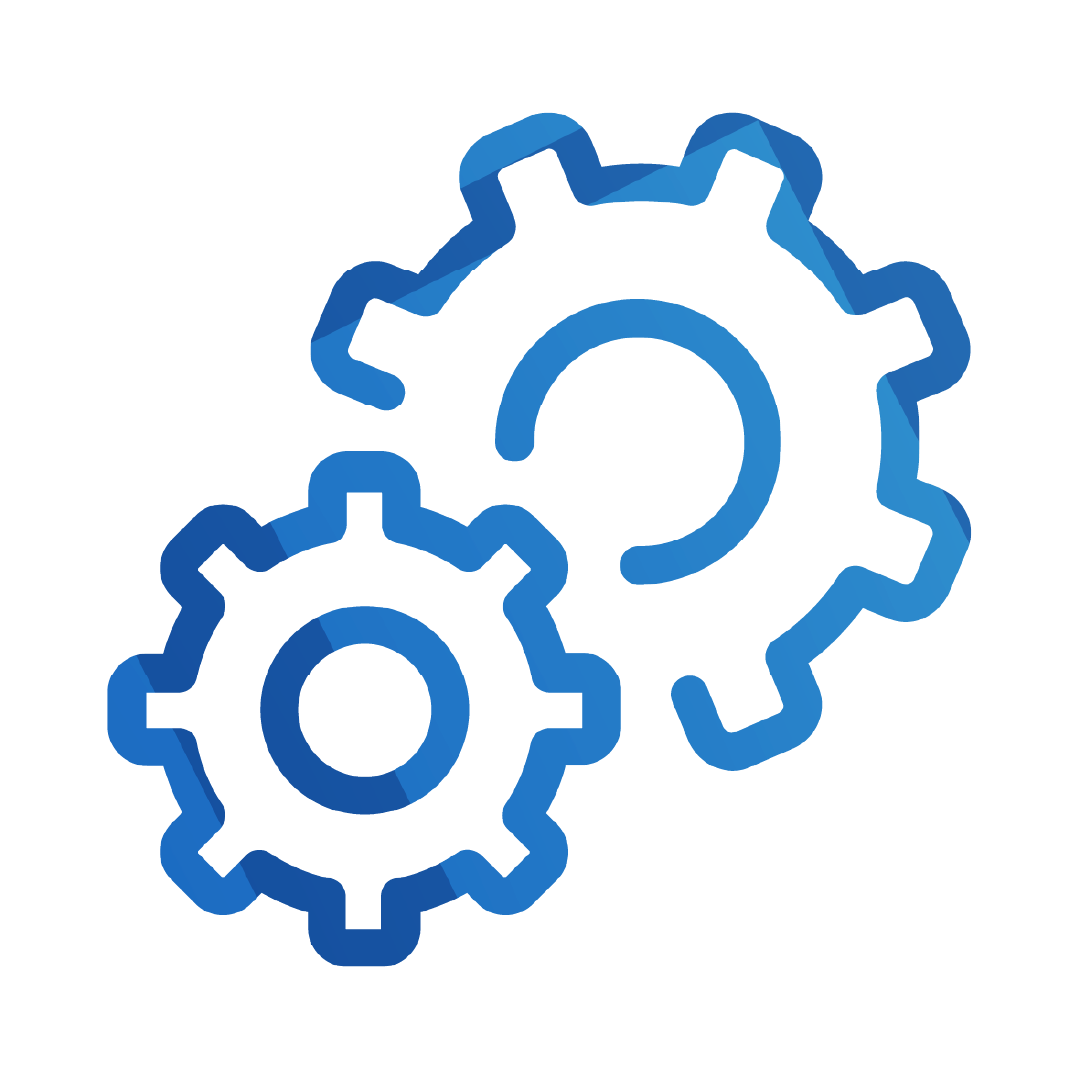
Streamline Operations
The one thing we hear over and over? Our customers didn’t realize how much more efficient Flex would make their operations. Flex reveals operational efficiencies our customers didn’t even know they needed.
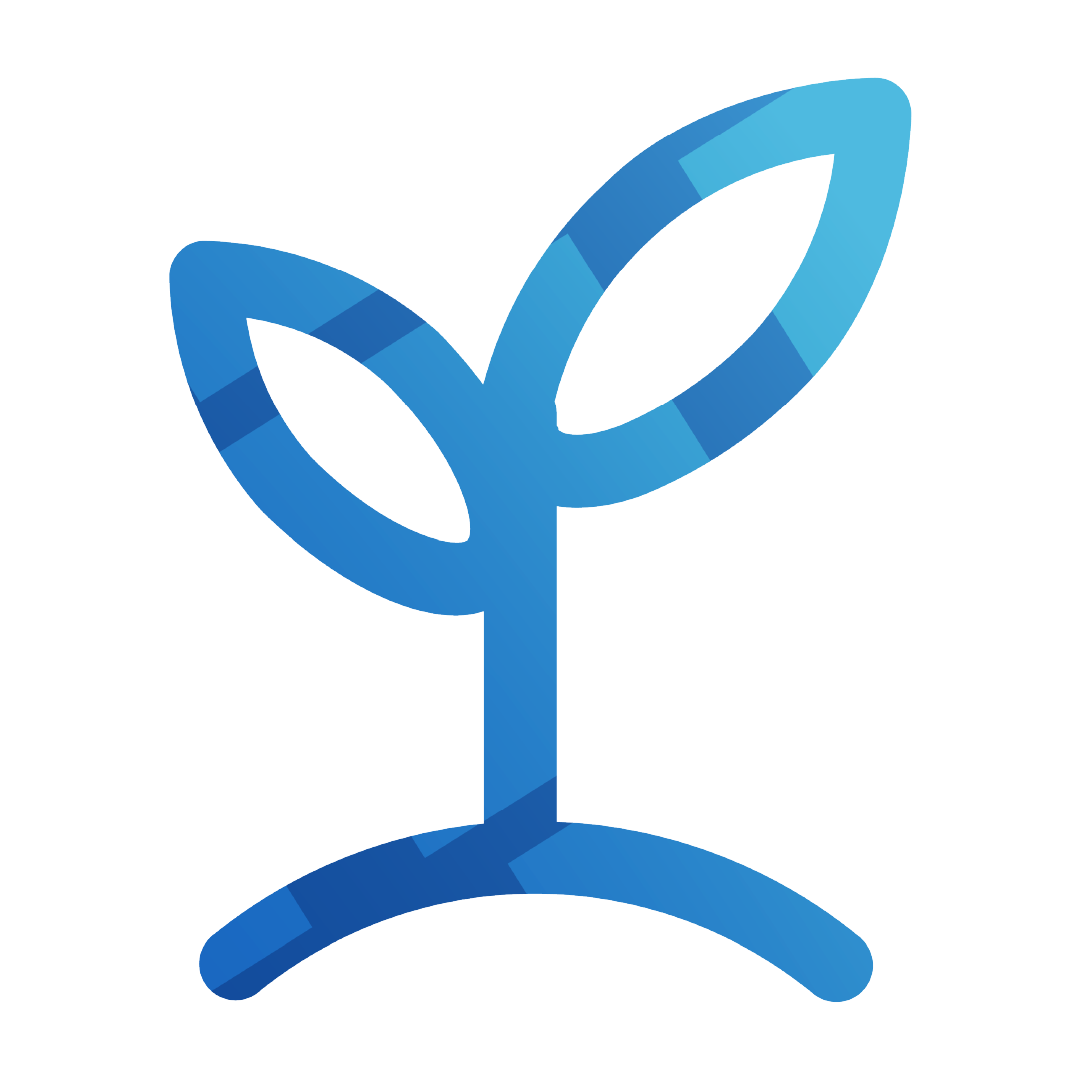
Expand Locations
Have you thought about expansion? Flex can help you. Haven’t thought about expansion? We’re guessing you might after using Flex. Flex creates a Center of Excellence that unlocks imaginations and invites new possibilities.
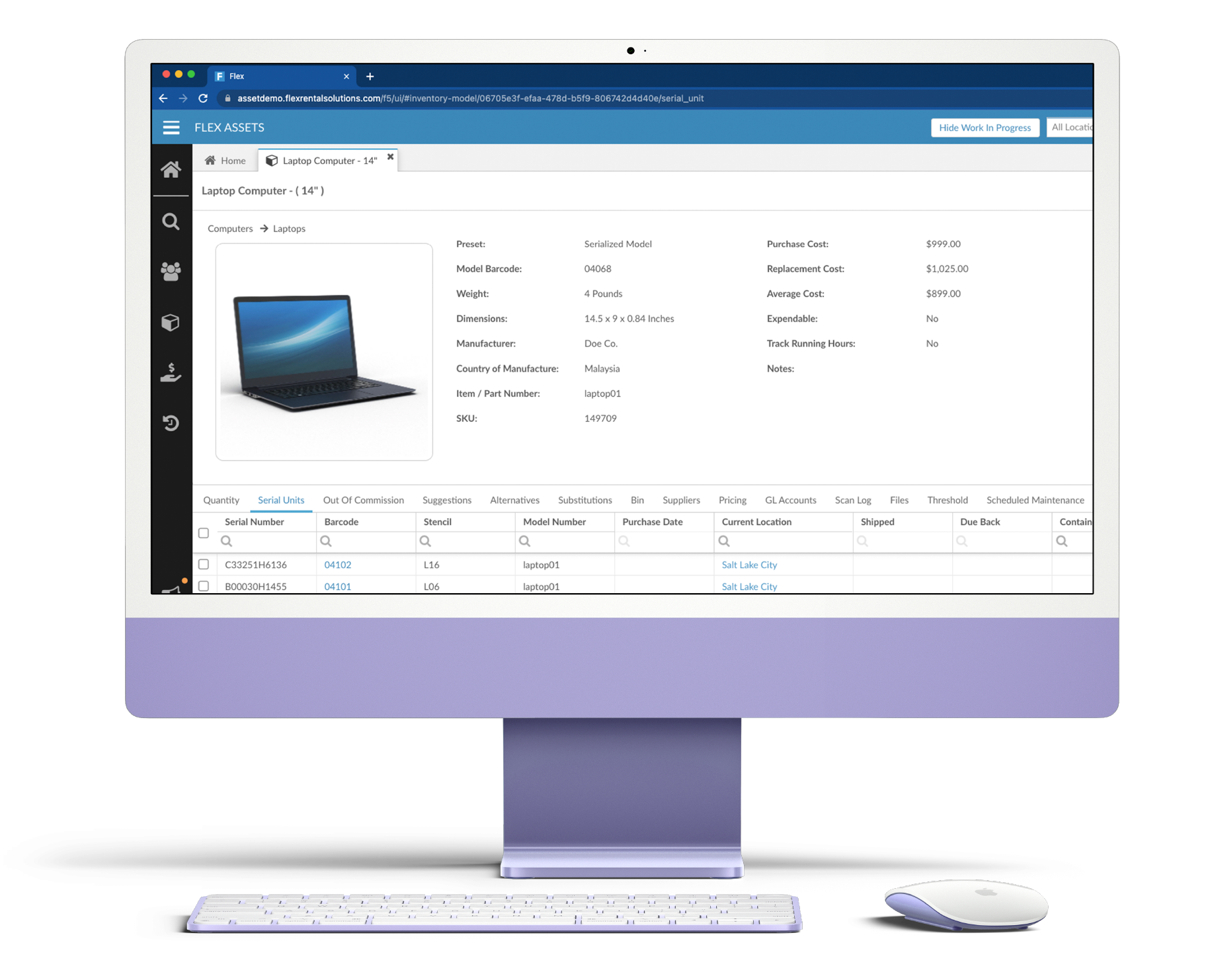
See what Flex can do for you
Reach out to us now, and let us know what you're looking to achieve.
Why Flex? Crafted from the most exacting experiences
We learned about the power and importance of proper asset management while touring with the biggest bands, working for United States Presidents, and waiting to hear, “And the Oscar goes to...” Then we put that experience to work to better manage assets in our own business. Now we offer you our expertise so you can efficiently administer your enterprise assets.

Quick Set Up
Get up and running in a matter of days not months.
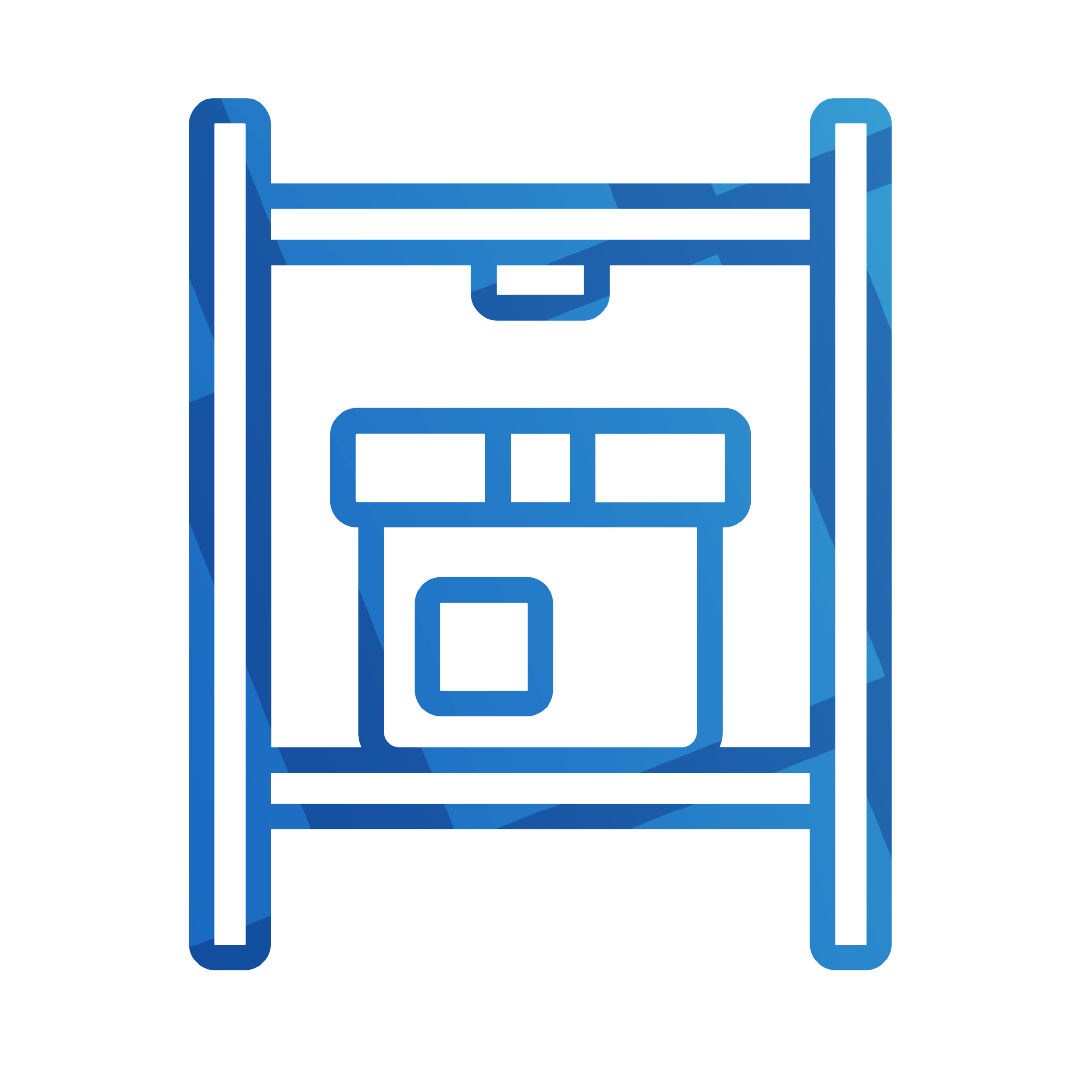
Improved Accuracy
Improve your data accuracy and stop relying on paperwork to track your data.

Easy to Use
Navigating your assets shouldn’t be hard and it isn’t with our intuitive app.

Best Practices
Benefit from the asset management lessons learned while supporting the biggest events in the world.

Client Success Stories
We pride ourselves on the fact that the best companies in the world use Flex.
Our success is your success. When you feel the pride, or the fulfillment, or the enthusiasm
for your success and milestones, we feel that too, because we are invested in you.